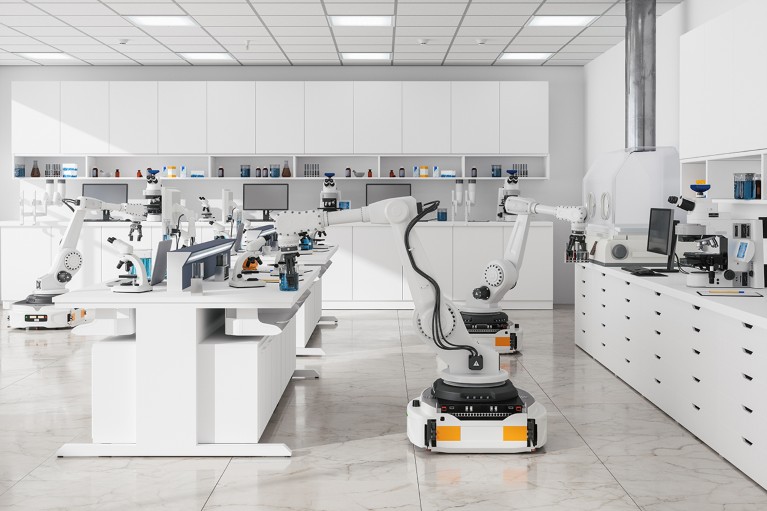
Artificial-intelligence models are able to translate an experimental method into code that runs a liquid-handling robot.Credit: Getty
Since July, researchers at Los Alamos National Laboratory in New Mexico have been assessing how the artificial intelligence (AI) model GPT-4o can assist humans with tasks in biological research. In the evaluations — which are being conducted to advance innovations in the biosciences, as well as to understand potential risks — humans ask GPT-4o various questions to help them achieve standard experimental tasks. These include maintaining and propagating cells in vitro; separating cells and other components in a sample using a centrifuge; and introducing foreign genetic material into a host organism.
In these assessments, researchers at Los Alamos are collaborating with OpenAI, the company in San Francisco, California, that developed GPT-4o. The tests are among a handful of efforts aiming to address potential biosafety and biosecurity issues posed by AI models since OpenAI made ChatGPT, a chatbot based on large language models (LLMs), publicly available in November 2022.
ChatGPT one year on: who is using it, how and why?
We argue that much more is needed.
Three of us investigate how scientific and technological innovations can affect public health and health security at the Johns Hopkins Center for Health Security in Baltimore, Maryland. Two of us research and develop solutions to public-policy challenges at the non-profit think tank RAND, which is headquartered in Santa Monica, California.
Although we see the promise of AI-assisted biological research to improve human health and well-being, this technology is still unpredictable and presents potentially significant risks. We urge governments to move faster to clarify which risks warrant most attention, and to determine what adequate testing and mitigation measures for these potential risks should entail. In short, we call for a more deliberate approach that draws on decades of government and scientific experience in reducing pandemic-scale risks in biological research1.
Experiments at speed
GPT-4o is a ‘multimodal’ LLM. It can accept text, audio, image and video prompts, and has been trained on vast quantities of these formats scraped from the Internet and elsewhere — data that almost certainly include millions of peer-reviewed studies in biological research. Its abilities are still being tested, but previous work hints at its possible uses in the life sciences. For instance, in 2023, Microsoft (a major investor in OpenAI) published evaluations of GPT-4, an earlier version of GPT-4o, showing that the LLM could provide step-by-step instructions for using the protein-design tool Rosetta to design an antibody that can bind to the spike protein of the coronavirus SARS-CoV-2. It could also translate an experimental protocol into code for a robot that can handle liquids — a capability that is “expected to greatly speed up the automation of biology experiments”2.
Also in 2023, researchers at Carnegie Mellon University in Pittsburgh, Pennsylvania, showed that a system using GPT-4, called Coscientist, could design, plan and perform complex experiments, such as chemical syntheses. In this case, the system was able to search documents, write code and control a robotic lab device3. And earlier this month, researchers at Stanford University in California and the Chan Zuckerberg Biohub in San Francisco introduced a Virtual Lab — a team of LLM agents powered by GPT4o that designed potent SARS-CoV-2 nanobodies (a type of antibody) with minimal human input4.
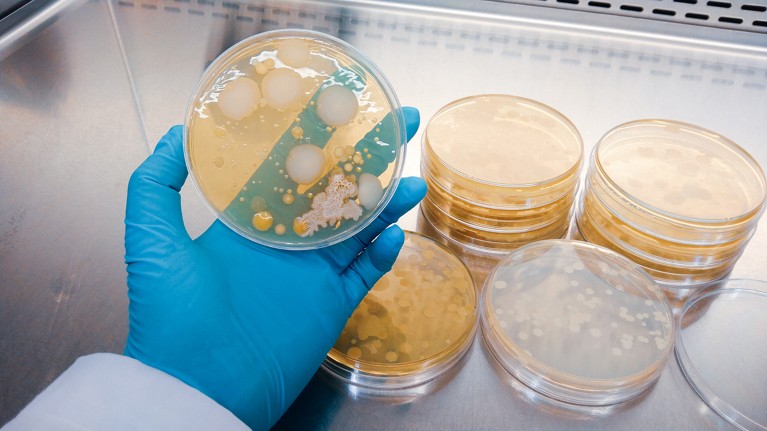
Automating lab protocols using AI systems would improve scalability and reduce costs.Credit: Nattapon Malee/Getty
OpenAI released GPT-4o in May, and is expected to release its successor, GPT-5, in the coming months. Most other leading AI companies have similarly improved their models. So far, assessments have focused mainly on individual LLMs operating in isolation. But AI developers expect combinations of AI tools, including LLMs, robotics and automation technologies, to be able to conduct experiments — such as those involving the manipulation, design and synthesis of drug candidates, toxins or stretches of DNA — with minimal human involvement.
These advances promise to transform biomedical research. But they could also bring significant biosafety and biosecurity risks5. Indeed, several governments worldwide have taken steps to try to mitigate such risks of cutting-edge AI models (see ‘Racing to keep up’). In 2023, for example, the US government secured voluntary commitments from 15 leading AI companies to manage the risks posed by the technology. Later that year, US President Joe Biden signed an Executive Order on the Safe, Secure, and Trustworthy Development and Use of Artificial Intelligence. Among other things, this requires companies to notify the government before they release models that are trained on “primarily biological sequence data” and that use “a quantity of computing power greater than 1023 integer or floating-point operations.”
The United Kingdom, the United States, Canada, Japan and Singapore have now established government institutes focused on AI safety to develop standards and tools for risk management. Other countries have committed to doing the same, with those five nations and Australia, France, Kenya and South Korea making up the founding members of an International Network of AI Safety Institutes, together with the European Union, which has established a safety unit in its AI Office.
These are impressive accomplishments in a short time frame, and should be supported. How much risk reduction has been achieved from all this activity, however, is unclear — in part because much of the work of these institutions has not yet been made public.
Safety testing
Separately from considerations of risk, some developers of AI models have tried to determine what factors affect their models’ performance the most. One leading hypothesis follows a scaling law: LLM performance improves with increases in model size, data-set size and computational power6. This is partly what influenced the US government’s decision to require AI companies to notify the Department of Commerce before releasing models that use a certain amount of computing power. But scaling laws will not reliably predict what capabilities could arise and when.
AI-generated images threaten science — here’s how researchers hope to spot them
In the meantime — in the absence of government policies on what risks urgently need addressing and how to mitigate them — companies such as OpenAI and Anthropic (also based in San Francisco) have followed evaluation protocols that they have developed in-house. (Many companies with AI systems, including Amazon in Seattle, Washington, Cohere in Canada, Mistral in Paris and xAI in San Francisco, have not yet made biosecurity evaluations of their models publicly available1.) In these cases, safety testing has entailed automated assessments, including those using multiple-choice questions (see go.nature.com/4tgj3p9); studies in which humans attempt to elicit harmful capabilities from the model being evaluated (known as red teaming; go.nature.com/3z4kg2p); and controlled trials in which individuals or groups are asked to perform a task with or without access to an AI model (uplift studies; go.nature.com/3unhgmr).
In our view, even when companies are conducting their own evaluations, such assessments are problematic. Often, they are too narrowly focused on the development of bioweapons. For instance, the technology company Meta conducted studies to see whether its open-source LLM Llama 3.1 could increase the proliferation of “chemical and biological weapons” (see go.nature.com/3reyqgs). Likewise, the AI company Anthropic has assessed whether its model Claude could answer “advanced bioweapon-relevant questions” (see go.nature.com/48u8tyj).
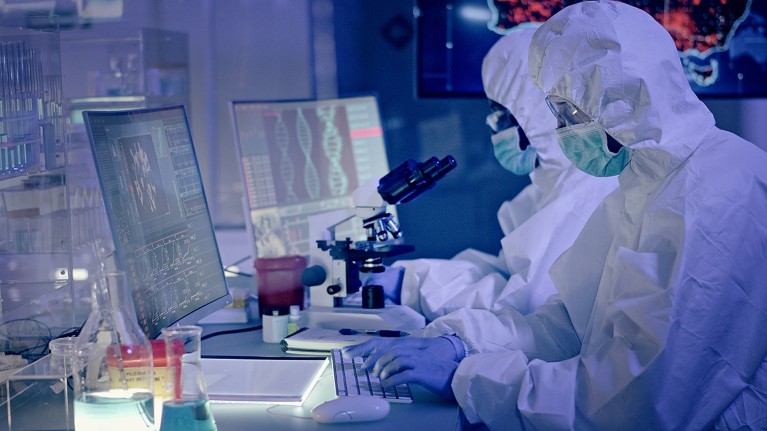
AI systems might enable the design of virus subtypes that evade immunity.Credit: Getty
The problem with this approach is that there is no publicly visible, agreed definition of ‘bioweapon’. When used in isolation, this term doesn’t differentiate between smaller-scale risks and large-scale ones. Various pathogens and toxins could plausibly be used as weapons, and many are listed in international non-proliferation agreements (see go.nature.com/3utzbw8). But few are likely to lead to the kinds of harm that could affect millions of people. Also, many pathogens, such as influenza and SARS-CoV-2, can cause severe societal disruption, but are not considered bioweapons.
Another issue is that evaluations have tended to focus too much on basic lab tasks. In the assessments being conducted by OpenAI in collaboration with Los Alamos researchers, for example, the capabilities being tested could be needed to develop something nefarious, such as a crop-destroying pathogen. But they are also essential steps for beneficial life-sciences research that do not — on their own — provide cause for alarm.
Added to all this, the evaluations conducted so far are resource-intensive and applicable mainly to LLMs. They generally involve a question-and-answer approach that requires humans to pose the questions or review a model’s answers. Finally, as mentioned earlier, evaluators need to examine how multiple AI systems operate in concert7 — something that is currently being requested by the US government but overlooked in industry, because companies are incentivized to test only their own models.
How to prioritize
So what does a better approach look like?
Given that resources are finite and progress in AI is rapid, we urge governments and AI developers to focus first on mitigating those harms that could result in the greatest loss of life and disruption to society. Outbreaks involving transmissible pathogens belong to this category — whether those pathogens affect humans, non-human animals or plants.
In our view, developers of AI models — working with safety and security experts — need to specify which AI capabilities are most likely to lead to this kind of pandemic-scale harm. A list of ‘capabilities of concern’ that various experts generally concur on, even if they disagree on some issues, offers a more robust starting point than does a list generated by individual companies or specialist academic groups.
Generative AI could revolutionize health care — but not if control is ceded to big tech
As a proof of principle, in June, we gathered 17 experts in AI, computational biology, infectious diseases, public health, biosecurity and science policy for a one-day hybrid workshop near Washington DC. The aim was to determine what AI-enabled capabilities in biological research would be most likely to enable a pandemic level of death and disruption — whether caused by a pandemic in humans or a widespread animal or crop disease. Views among workshop participants differed. Still, the majority of the group members rated 7 AI capabilities from a list of 17 as being “moderately likely” or “very likely” to enable new global outbreaks of human, animal or plant pathogens. These are:
Optimizing and generating designs for new virus subtypes that can evade immunity. A study8 showing that an AI model can generate viable designs for subtypes of SARS-CoV-2 that can escape human immunity was published in Nature in 2023.
Designing characteristics of a pathogen to enable its spread within or between species. AI systems might allow the design of proteins, genes or genomes that generate characteristics in pathogens that affect their transmissibility. So far, human-induced genetic alterations to pathogens have not been evolutionarily durable, but AI developers are working on models that can design genetic changes that persist9.
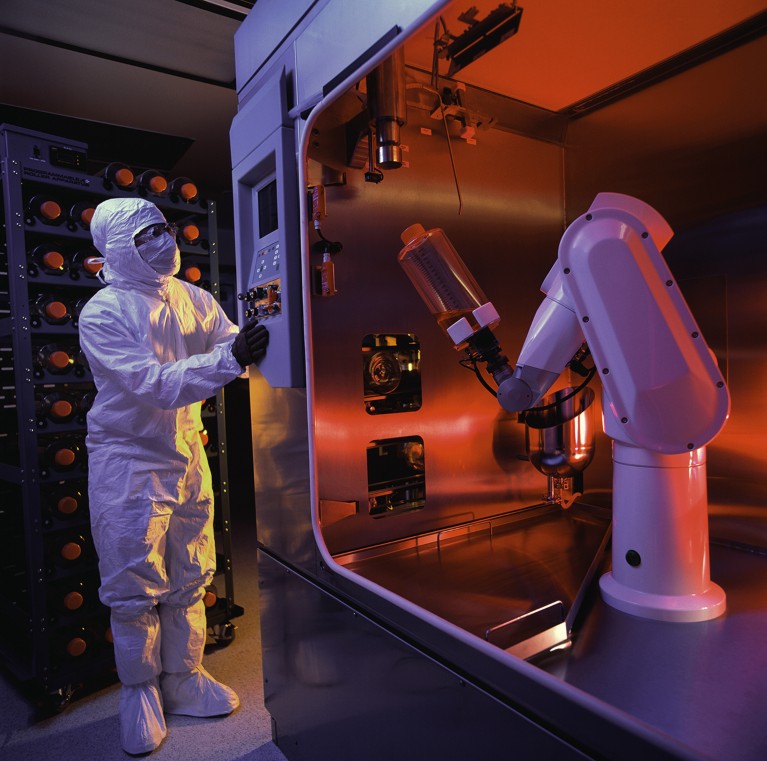
Combining AI tools with robotics will enable experiments involving biohazards to be conducted with minimal human involvement.Credit: Bill Varie/Getty
Generating vast amounts of data on traits that determine how easily viruses can be transmitted — which could, in turn, be used to train other AI models. Currently, determining which characteristics help a viral pathogen to transfer from one cell to another, or from one host to another, involves time-intensive wet-lab methods. Industry and academic researchers are trying to develop autonomous robotics and other AI systems that can perform some of these steps.
Assisting or completing protocols for the de novo synthesis of human, animal or plant pathogens. Commercial entities such as contract research organizations provide research services on a contractual basis, but the step-by-step protocols they perform generally involve human labour. There is now interest in automating some of this using AI systems and agents to improve scalability and reduce costs2,10.
Designing genes, genetic pathways or proteins that convert non-human animal pathogens into human pathogens. Most infectious diseases in humans arise from non-human animals. (Some diseases that began in animals can mutate into strains that infect only humans, as happened with HIV.) So far, it has been hard to predict which genes, strains or proteins increase the likelihood of a pathogen being transferred from an animal to a human. To improve such predictions, AI developers might build systems that can integrate vast quantities of pathogen genomic data with information on the traits that affect transmissibility. (Currently, there are insufficient training data to do this, and collecting these poses its own risks.)
Science and the new age of AI: a Nature special
Designing proteins, genes or genetic pathways in pathogens so that they selectively harm certain human populations. AI systems that integrate human genomic data with pathogen data might be able to discern — for good or harm — why particular human populations are more or less susceptible to a pathogen.
Modelling how diseases spread using pathogen genomic data. Epidemiological modelling refers to the computational simulation of disease outbreaks, based on the characteristics of the pathogen and the human population. AI could make such forecasting easier and more accurate. Future AI systems might even be able to provide rough estimates on spread on the basis of pathogen genomic information alone.
Guidance needed
All of these AI capabilities are being studied for their potential beneficial applications — for instance, to guide the design of vaccines. Government policies that preserve such benefits while mitigating risks, or that provide guidance on what the safer alternatives might be, are therefore crucial.
But only once it is clear which AI capabilities pose pandemic-scale biosafety and biosecurity risks can effective evaluations for them be developed. In other words, there must be a strong correlation between whatever capability is being tested and the likelihood of a high-risk event occurring. If such a capability is then detected through safety testing, targeted efforts can be made to reduce the risks.
What ChatGPT and generative AI mean for science
Attempts to elicit harmful capabilities from AI models during a testing phase could generate different results depending on the approach used and the level of effort made. To be effective, then, tests of capabilities must be sufficiently reliable. Also, evaluations should be undertaken by specialists who have a deep knowledge of the technology, but who are not beholden to the company that developed the AI system or systems being evaluated. Currently, this is a considerable difficulty, because those who best understand how to test AI models were often involved in their development. But new government institutions, such as the US and UK AI safety institutes, can build independent expertise — as long as they continue to be adequately funded and supported. These two institutes have already recruited leaders from top AI companies.
Some have argued — reasonably — that the time and resources currently required for AI biosecurity testing puts such tests out of reach for smaller AI companies and academic labs. In its recent GPT-4o evaluation, OpenAI worked with more than 100 external red-teamers to draw out the model’s potential harmful capabilities. If more of the steps involved become automated, however, safety tests of AI systems could become simple, routine and affordable. Such a shift has occurred in other fields such as cybersecurity, in which software tools have replaced human hackers.
On 20–21 November, representatives from countries that have established AI Safety institutes, or that are committed to doing so, are gathering in San Francisco to hash out how companies might — in practice — develop AI systems in a safe and ethical way. And in February, heads of state and industry leaders will discuss how to build trust in AI “based on an objective scientific consensus on safety and security issues” at the global AI Action Summit in Paris.
All of this is encouraging. But the first step is to build an objective scientific consensus through proactive processes that engage diverse — and independent — experts.